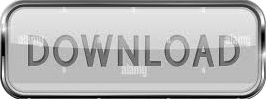
Moreover, up to 1.65X performance speedup with an average of 1.19X are observed.Ī large class of IoT applications read sensors, execute application logic, and actuate actuators. Based on this observation, we propose an enhanced learning-based approach that relaxes such equivalence requirements but supplements them with constraining conditions to make them semantically equivalent when such rules are applied.Įxperimental results on SPEC CINT2006 show that the proposed approach can improve the dynamic coverage of the translation from 55.7% to 69.1% and the static coverage from 52.2% to 61.8%, compared to the original approach. It excludes many potentially high-quality rule candidates from being included and applied. In this paper, we first give an in-depth analysis on the constraints of prior learning-based methods and observe that the equivalence requirements are often unduly restrictive. But, they still suffer from some shortcomings.
#Lightning atc verification#
To solve this problem, a learning-based approach was recently proposed to automatically learn semantically-equivalent translation rules, and symbolic verification is used to prove the semantic equivalence of such rules.
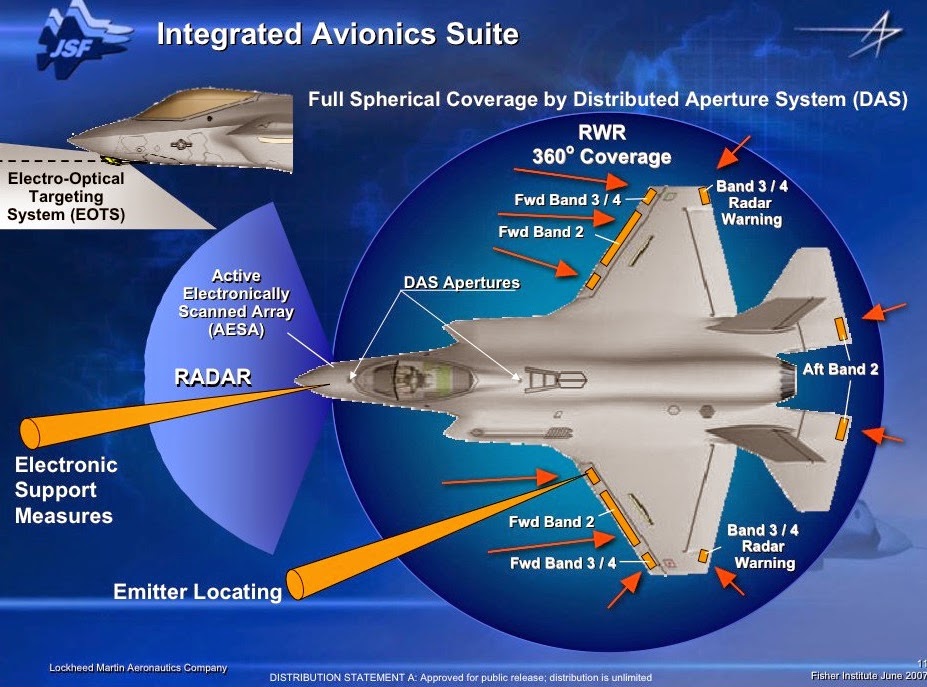

However, most translation rules in existing DBT systems are created manually with high engineering efforts and poor quality. To achieve good performance, it is important for a DBT system to be equipped with high-quality translation rules. Latency on an unloaded system is about 4 times higher than a Linux NFS server backed by NVMe, reflecting the cost of replication.ĭynamic binary translation (DBT) is a key system technology that enables many important system applications such as system virtualization and emulation. The system throughput can be predicted by comparing an estimate of the network bandwidth needed for replication to the network bandwidth provided by the hardware. We use a non-scalable Paxos-replicated hash table to store configuration information required to bootstrap the system. The presentation servers maintain no persistent local state, with any state kept in the B-tree, making it easy to scale up and failover the presentation servers. The entire system is programmed using a nonblocking lock-free programming style. The filesystem provides snapshots by using versioned key-value pairs. The B-tree holds the filesystem metadata. By using self-validating B-tree nodes and performing all Btree housekeeping operations as separate transactions, each key in a B-tree transaction requires only one page in the underlying block transaction. On top of the block store, we built a scalable B-tree that provides linearizable multikey limited-size transactions. Using a pipelined Paxos implementation, we implemented a scalable block store that provides linearizable multipage limited-size transactions. These lessons can be of value to the designers and operators of IaaS facilities in general, systems testbeds in particular, and users who have a stake in understanding how these systems are built.įile Storage Service (FSS) is an elastic filesystem provided as a managed NFS service in Oracle Cloud Infrastructure.

Our second set of lessons comes from looking at the ways that algorithms used "under the hood," such as resource allocation, have important-and sometimes unexpected-effects on user experience and behavior.

The first set comes from analysis of data regarding the use of CloudLab: how users interact with it, what they use it for, and the implications for facility design and operation. From this experience, we draw lessons organized around two themes. We present our experiences designing CloudLab and operating it for four years, serving nearly 4,000 users who have run over 79,000 experiments on 2,250 servers, switches, and other pieces of datacenter equipment. In this paper, we cover one such facility, CloudLab, which supports systems research by providing raw access to programmable hardware, enabling research at large scales, and creating a shared platform for repeatable research. Given the highly empirical nature of research in cloud computing, networked systems, and related fields, testbeds play an important role in the research ecosystem.
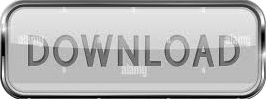